1. 什么是 TensorRT
TensorRT 是一个 C++ 库,主要用在 NVIDIA GPU 进行高性能的推理加速上,提供了 C++ API 和 Python API 用于集成。
TensorRT 支持的主流深度学习框架有:
- Caffe,TensorRT 可以直接读取 prototxt 格式
- TensorFlow,需要将 TensorFlow 的 pb 转换为 uff 格式
- PyTorch,需要将 PyTorch 的 pth 格式转换为 onnx 格式
- MXNet,需要将 MxNet 的 params 格式转换为 onnx 格式
TensorRT 是专门针对 NVIDIA GPU 的推理引擎,并不适用于其他厂商。
另外,TensorRT 也不是完全开源的,核心的运行时库 libnvinfer.so 是闭源的,只有相关的周边库、API 才是开源的。
2. TensorRT 优化原理
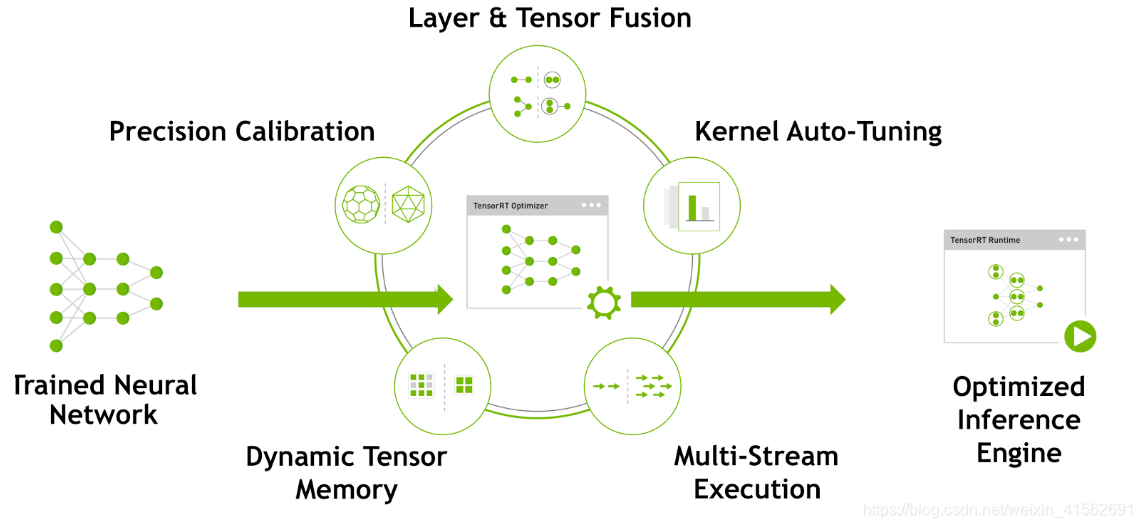
在推理时,大量时间浪费在 CUDA 核心的启动和每层输入输出的读写上,造成内存带宽瓶颈和 GPU 资源的浪费。
TensorRT 可通过层间的横向、纵向融合成一个 CBR (Convolution-BatchNorm-ReLU) 层,模型层级少,GPU 核心利用率高,从而提高推理性能。
在训练模型,网络中的参数精度通常为 FP32,32 位浮点数的推理性能很低,占用大量的 GPU 内存。但推理时,由于不需要反向传播,可以适当降低参数精度,从而提高推理性能。
TensorRT 对这一量化过程提供了自动化的支持,能够减少模型精度损失的同时,提高推理性能。
TensorRT 能根据不同显卡架构、SM 数量、内核频率等,选择最合适的的策略和计算方式。
TensorRT 在运行时,动态分配显存,以提高显存的利用率,支持更大的网络。
TensorRT 支持在同一个 GPU 上执行多个 Stream,同时操作,提高 GPU 的利用率。
3. 模型转换为 TensorRT
3.1 PyTorch
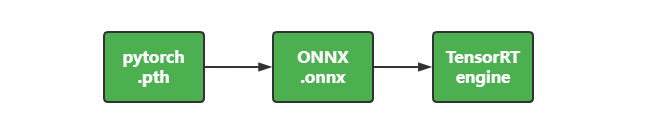
PyTorch 采用的是动态的计算图。将 PyTorch 模型转为 ONNX 时,需要调用 PyTorch 的 torch.onnx.export
函数。将 PyTorch 模型转为 ONNX 时,有两种方法:
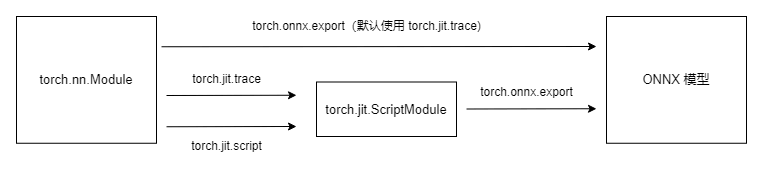
通过实际运行一遍模型的方法导出模型的静态图,但无法识别出模型中的控制流,如循环等。导出的思路就是让模型进行一次推理,记录下计算图。trace 导出的是静态图,推理引擎执行的效率更高。
通过解析模型来记录所有的计算过程。
3.2 TensorFlow

TensorFlow 采用的是静态的计算图,本身具有图的完整结构。
由于 ckpt 格式有很多冗余的信息,pb 格式体积更小,通常会先将模型转为 pb。而 pb 的计算图优化不如 uff 好,效率低。因此,又会先转 uff,再转 TensorRT 。
4. PyTorch 转 ONNX 转 TensorRT
1
| docker run -v $PWD:/runtime shaowenchen/huggingface-cli download --resume-download --local-dir-use-symlinks False THUDM/ChatGLM2-6B --local-dir ChatGLM2-6B
|
在 Pytorch 转换为 ONNX 格式时,只需要执行 torch.onnx.export
函数即可。但经常会遇到一些,算子不支持、RuntimeError、ShapeError 等问题,这就需要对模型参数、算子进行调试。网上会有些分享,并经过验证的脚本,可以用来转换指定的一些模型。
1
| git clone https://github.com/luchangli03/export_llama_to_onnx
|
1
| pip install numpy transformers torch==2.1 onnx -i https://pypi.tuna.tsinghua.edu.cn/simple
|
1
2
3
4
5
6
7
8
9
| python3 export_llama_to_onnx/export_chatglm2.py -m ChatGLM2-6B -o ./ChatGLM2-6B-onnx -p float16 -d cuda
begin load model from chatglm2-6b
Loading checkpoint shards: 100%|███████████████████████████████████████████████████████████████████████████████████████████████████████████████████████████████████████████| 7/7 [00:19<00:00, 2.78s/it]
convert model to float16
convert model to cuda
finish load model from chatglm2-6b
begin export chat_glm_model
layer_num: 28
|
在 ./ChatGLM2-6B-onnx
目录下会生成大量 .onnx
后缀的文件。
1
2
3
4
5
6
7
8
9
10
| import onnx
try:
onnx.checker.check_model("./ChatGLM2-6B-onnx/chat_glm_model.onnx")
onnx_model = onnx.load("./ChatGLM2-6B-onnx/chat_glm_model.onnx")
print("ONNX Version:", onnx_model.ir_version)
except Exception as e:
print("Model incorrect: {}".format(e))
else:
print("Model correct")
|
此时应该输出
1
2
| ONNX Version: 8
Model correct
|
1
| netron --host 0.0.0.0 -p 8080 ./ChatGLM2-6B-onnx/chat_glm_model.onnx
|
访问 http://localhost:8080/ 查看模型结构。
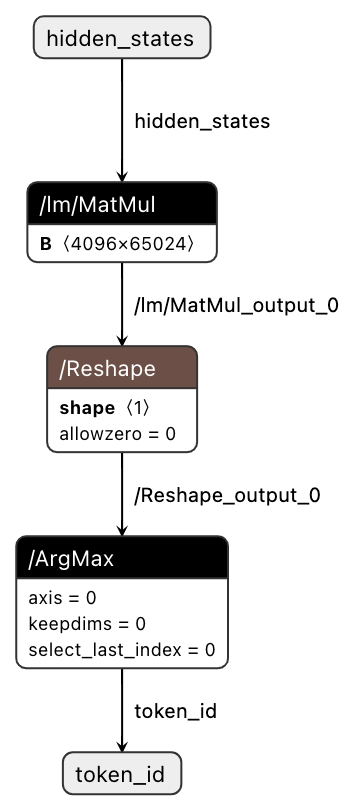
进入容器编译环境
1
| docker run --gpus device=all -v $PWD:/workspace -it --rm nvcr.io/nvidia/tensorrt:23.12-py3 bash
|
开始转换
1
2
3
4
5
6
7
8
9
10
11
12
13
14
| trtexec --onnx=./ChatGLM2-6B-onnx/chat_glm_model.onnx --saveEngine=./ChatGLM2-6B-trt-engines/chat_glm_model.engine
[02/04/2024-07:25:07] [I] === Performance summary ===
[02/04/2024-07:25:07] [I] Throughput: 63.7494 qps
[02/04/2024-07:25:07] [I] Latency: min = 15.8738 ms, max = 16.2778 ms, mean = 15.935 ms, median = 15.9253 ms, percentile(90%) = 15.9812 ms, percentile(95%) = 16.0046 ms, percentile(99%) = 16.1168 ms
[02/04/2024-07:25:07] [I] Enqueue Time: min = 2.09204 ms, max = 6.43628 ms, mean = 3.0315 ms, median = 2.94957 ms, percentile(90%) = 3.47656 ms, percentile(95%) = 4.021 ms, percentile(99%) = 4.52615 ms
[02/04/2024-07:25:07] [I] H2D Latency: min = 0.18042 ms, max = 0.573242 ms, mean = 0.206923 ms, median = 0.194153 ms, percentile(90%) = 0.258423 ms, percentile(95%) = 0.273682 ms, percentile(99%) = 0.375793 ms
[02/04/2024-07:25:07] [I] GPU Compute Time: min = 15.5573 ms, max = 15.6531 ms, mean = 15.6016 ms, median = 15.6018 ms, percentile(90%) = 15.6212 ms, percentile(95%) = 15.6265 ms, percentile(99%) = 15.6391 ms
[02/04/2024-07:25:07] [I] D2H Latency: min = 0.12439 ms, max = 0.132263 ms, mean = 0.12646 ms, median = 0.126465 ms, percentile(90%) = 0.12793 ms, percentile(95%) = 0.128418 ms, percentile(99%) = 0.131042 ms
[02/04/2024-07:25:07] [I] Total Host Walltime: 3.04316 s
[02/04/2024-07:25:07] [I] Total GPU Compute Time: 3.02671 s
[02/04/2024-07:25:07] [I] Explanations of the performance metrics are printed in the verbose logs.
[02/04/2024-07:25:07] [I]
&&&& PASSED TensorRT.trtexec [TensorRT v8601] # trtexec --onnx=./ChatGLM2-6B-onnx/chat_glm_model.onnx --saveEngine=./ChatGLM2-6B-trt-engines/chat_glm_model.engine
|
会有一些性能测试的结果信息,P95、P99 等以供参考。
1
2
3
| ls -alh ./ChatGLM2-6B-trt-engines/chat_glm_model.engine
-rw-r--r-- 1 root root 24G Feb 4 07:24 ./ChatGLM2-6B-trt-engines/chat_glm_model.engine
|
但对于大模型来说,使用 TensorRT-LLM 进行转换会是一个更好的选择,不仅仅是转换的成功率、效率,还有转换之后也更好部署与 Triton 进行集成。
5. TensorRT-LLM 转 TensorRT
1
| docker run --gpus device=0 -v $PWD:/app/tensorrt_llm/models -it --rm shaowenchen/nvidia-tensorrt-llm:v0.7.1 bash
|
1
2
3
4
5
6
7
8
9
10
11
12
13
14
15
16
17
18
19
20
21
22
| python examples/chatglm/build.py --model_dir ./models/ChatGLM2-6B \
--model_name chatglm2_6b \
--dtype float16 \
--parallel_build \
--use_inflight_batching \
--enable_context_fmha \
--use_gemm_plugin float16 \
--use_gpt_attention_plugin float16 \
--output_dir ./models/ChatGLM2-6B-trt-engines
[02/05/2024-02:50:58] [TRT] [I] [MemUsageChange] TensorRT-managed allocation in engine deserialization: CPU +0, GPU +11908, now: CPU 0, GPU 11908 (MiB)
[02/05/2024-02:50:58] [TRT-LLM] [I] Activation memory size: 210.50 MiB
[02/05/2024-02:50:58] [TRT-LLM] [I] Weights memory size: 11909.66 MiB
[02/05/2024-02:50:58] [TRT-LLM] [I] Max KV Cache memory size: 448.00 MiB
[02/05/2024-02:50:58] [TRT-LLM] [I] Estimated max memory usage on runtime: 12568.16 MiB
[02/05/2024-02:50:58] [TRT-LLM] [I] Serializing engine to models/ChatGLM2-6B-trt-engines/chatglm2_6b_float16_tp1_rank0.engine...
[02/05/2024-02:51:03] [TRT-LLM] [I] Engine serialized. Total time: 00:00:04
[02/05/2024-02:51:03] [TRT] [I] Serialized 59 bytes of code generator cache.
[02/05/2024-02:51:03] [TRT] [I] Serialized 35129 bytes of compilation cache.
[02/05/2024-02:51:03] [TRT] [I] Serialized 315 timing cache entries
[02/05/2024-02:51:03] [TRT-LLM] [I] Timing cache serialized to model.cache
[02/05/2024-02:51:05] [TRT-LLM] [I] Total time of building all 1 engines: 00:00:55
|
1
2
3
4
5
6
7
8
| python examples/run.py --input_text "世界上第三高的山峰是哪座?" \
--max_output_len=200 \
--tokenizer_dir ./models/ChatGLM2-6B \
--engine_dir=./models/ChatGLM2-6B-trt-engines/
[02/05/2024-02:55:55] [TRT-LLM] [W] Found pynvml==11.4.1. Please use pynvml>=11.5.0 to get accurate memory usage
Input [Text 0]: "世界上第三高的山峰是哪座?"
Output [Text 0 Beam 0]: "世界上第三高的山峰是干城章嘉峰,它位于印度洋上的马达加斯加岛。这座山峰高5895米,被誉为“火山中的活火山”
|
6. 总结
TensorRT 是 NVIDIA 推出的用于在 GPU 上进行高性能推理加速的 C++ 库,通过层合并、量化、Kernel 优化等技术提升推理性能。
TensorRT 支持主流深度学习框架模型的转化,如 PyTorch 到 ONNX 然后到 TensorRT,转换过程中可能需要处理某些算子不支持的问题。
PyTorch 可以通过 trace 或 script 两种方法导出 ONNX 模型。
TensorFlow 模型可以通过 ckpt->pb->uff->TensorRT 的转换链实现转化。
TensorRT-LLM 是 NVIDIA 推出的用于大模型推理加速的 TensorRT 解决方案,如果是大模型使用 TensorRT-LLM 是一个更好的选择。